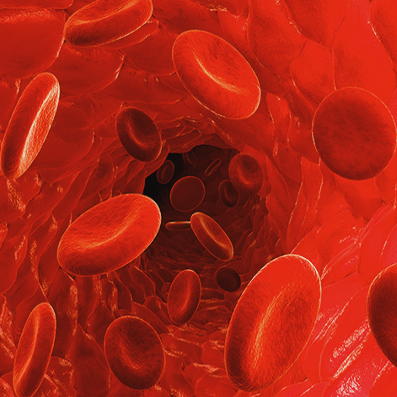
Proteins are often called the building blocks of life. In reality, proteins are more like flexible tumbleweeds-highly sophisticated structures with “Spikes” and branches protruding from a central frame-that morph and change with their environment.
It also makes understanding protein behavior and developing drugs that interact with proteins a challenge.
While recent AI breakthroughs in the prediction of protein structures are a huge advance 50 years in the making, they still only offer snapshots of proteins.
To capture whole biological processes-and identify which lead to diseases-we need predictions of protein structures in multiple “Poses” and how each of these poses changes a cell’s inner functions.
Thanks to a new protein atlas published this month in Nature, we now have a great start.
A collaboration between MIT, Harvard Medical School, Yale School of Medicine, and Weill Cornell Medical College, the study focused on a specific chemical change in proteins-called phosphorylation-that’s known to act as a protein on-off switch, and in many cases, lead to or inhibit cancer.
To Sean Humphrey and Elise Needham, doctors at the Royal Children’s Hospital and the University of Cambridge, respectively, who were not involved in the work, the atlas may also begin to help turn static AI predictions of protein shapes into more fluid predictions of how proteins behave in the body.
Let’s Talk About PTMs. After they’re manufactured, the surfaces of proteins are “Dotted” with small chemical groups-like adding toppings to an ice cream cone. These toppings either enhance or turn off the protein’s activity.
Protein tags in neurons drive brain development; other tags plant red flags on proteins ready for disposal. PTMs essentially transform proteins into biological microprocessors.
PTMs often dramatically change the structure and function of proteins, and in some cases, they could contribute to Alzheimer’s, cancer, stroke, and diabetes.
For Elisa Fadda at Maynooth University in Ireland and Jon Agirre at the University of York, it’s high time we incorporated PTMs into AI protein predictors like AlphaFold.
While AlphaFold is changing the way we do structural biology, they said, “The algorithm does not account for essential modifications that affect protein structure and function, which gives us only part of the picture.”
This PTM adds a chemical group, phosphate, to specific locations on proteins. The protein hotspots for phosphorylation are well-known: two amino acids, serine and threonine.
Based on their findings, the team grouped the kinases into 38 different motif-based classes, each with an appetite for a particular protein target.
In theory, the kinases can catalyze over 90,000 known phosphorylation sites in proteins. The dataset, when fed into AlphaFold, RoseTTAFold, their variants, or other emerging protein structure prediction algorithms, could help them better predict how proteins dynamically change shape and interact in cells.
In other words-we have no idea how these powerful proteins work inside the body.
“This possibility should motivate researchers to venture ‘into the dark’, to better characterize these elusive proteins,” said Humphrey and Needham.