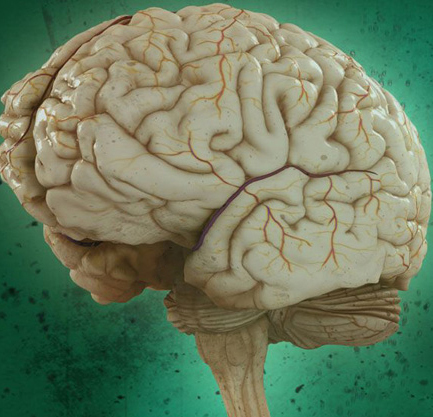
Artificial intelligence has advanced significantly, yet there remains a crucial challenge: accurately testing for human-like intelligence in AI systems. Recent initiatives by leading AI players, such as Scale AI and the Center for AI Safety, have spotlighted this issue by launching “Humanity’s Last Exam.” This initiative invites the public to devise questions that probe the capacities of large language models (LLMs) like Google Gemini and OpenAI’s o1. The goal is to pinpoint how closely these AI systems approach “expert-level AI systems,” through what’s being touted as the largest coalition of experts in history
Current AI models excel in narrow domains like law and mathematics, suggesting they possess advanced intelligence. However, there’s a concern that these systems might not truly understand content but rather recall pre-learned answers from their vast training data, which encompasses a significant portion of the internet. As AI continues to consume and learn from this expansive dataset, a future where AI has “read” everything ever written seems imminent, possibly by 2028.
One emerging challenge is the potential for “model collapse,” where the increasing proliferation of AI-generated content online could degrade the quality of AI training data over time. This might necessitate new strategies, such as integrating real-world experiences similar to how Tesla uses real-world driving data to train its AI. Additionally, experts are exploring ways to use human-centric data from devices like Meta’s smart glasses to enhance AI training.
Despite these efforts, defining and measuring intelligence, especially artificial general intelligence (AGI) that matches or exceeds human capabilities, remains complex. Traditional IQ tests have been criticized for not capturing the full spectrum of human intelligence, and similarly, existing AI benchmarks may be too narrow or task-specific. For instance, the chess-playing AI Stockfish excels at chess to a degree far beyond any human but cannot comprehend language or perform other intellectual tasks, highlighting the need for more comprehensive measures of AI capabilities.
New approaches are being developed to better assess AI intelligence. One promising direction is the “abstraction and reasoning corpus” (ARC), which tests AI’s ability to infer and apply abstract rules in novel scenarios, offering a more robust measure of genuine intelligence. However, even with such innovative approaches, the journey to truly understand and measure AI intelligence continues, marked by both technological advances and philosophical inquiries.