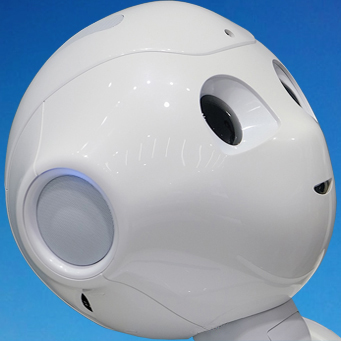
Our adaptability to an ever-changing world is a superpower that currently escapes most AI agents. Even the most sophisticated AI agents break down-or require untenable amounts of computing time-as they juggle conflicting goals.
Although able to learn from its mistakes, the AI struggles to find the right balance when challenged with multiple opposing goals simultaneously. In a new study published in PNAS, the team took a page from cognitive neuroscience and built a modular AI agent.
Rather than a monolithic AI-a single network that encompasses the entire “Self”-the team constructed a modular agent, each part with its own “Motivation” and goals but commanding a single “Body.” Like a democratic society, the AI system argues within itself to decide on the best response, where the action most likely to yield the largest winning outcome guides its next step.
By deconstructing an AI agent, the research doesn’t just provide insight into smarter machine learning agents.
In a sense, the monolithic agent is a unified brain that tries to maximize the best outcome after simultaneously processing all resources in tandem.
The opponent: modular AI. Like an octopus with semi-autonomous limbs, the AI agent is broken down into sub-agents, each with its own goals and feedback.
To make it a fair fight, each module is also trained with DQL. The separate “Brains” observe their surroundings and learn to select the best option-but only tailored to their own goals.
The solution with the potential optimal outcome is then selected, piloting the AI agent on to its next choice. Each AI agent roams around a two-dimensional grid that has different types of resources hidden in some regions. The goal is to keep the agent’s four stats at their set level, with each gradually decreasing over time.
If the agent had a low ‘hunger’ stat, it could collect the ‘food’ resource by moving to the location of that resource,” explained the team.
The monolithic agent readily maintained its four stats after 30,000 training steps, though it went through a period of overshooting and undershooting until reaching the targeted goals.
By 5,000 learning steps, the agent had already captured an understanding of the “State of the world.”
Unlike previous methods for modular systems that divide and conquer to move towards a final goal, here the AI represents a more holistic social relationship-one in which some modules gain and some lose through a constant state of internal competition.
Because the AI agent’s “Body” is guided only by the winning module, the losing ones have to go along with a decision they didn’t agree with and are forced into a new reality.
This adaptability further shone when the team challenged both AI agents in changing environments. The modular AI quickly picked up on the changes and adapted to them, whereas the monolithic agent performed far worse. In another test the team turned up the dial, requiring the AI agents to simultaneously maintain eight factors rather than the original four.
The modular agent rapidly adapted to hunt down resources to maintain its goals. In contrast, the monolithic agent again struggled, taking far longer to return to the desired levels for each of its stats.
Similar to previous work, the modular modules show that it’s possible to have a single AI agent learn separate and easier sub-problems in parallel in a way that’s relatively decentralized in terms of data processing.