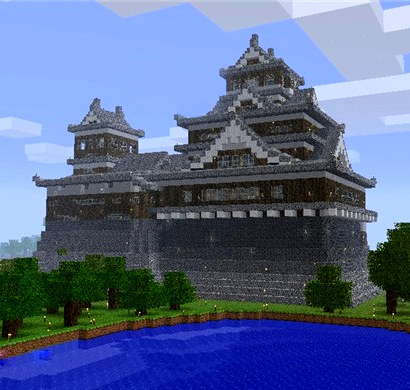
All kinds of machines, from industrial robots to drones, are gathering a growing amount of their training data and practice hours inside virtual worlds.
According to Gautham Sholingar, a senior manager at Nvidia focused on autonomous vehicle simulation, one key benefit is accounting for obscure scenarios for which it would be nearly impossible to gather training data in the real world.
“Without simulation, there are some scenarios that are just hard to account for. There will always be edge cases which are difficult to collect data for, either because they are dangerous and involve pedestrians or things that are challenging to measure accurately like the velocity of faraway objects.
That’s where simulation really shines,” he told Singularity Hub.
Industrial use of simulation has been around for decades, something Sholingar pointed out, but a convergence of improvements in computing power, the ability to model complex physics, and the development of the GPUs powering today’s graphics indicate we may be witnessing a turning point in the use of simulated worlds for AI training.
In 2021, the company launched Omniverse, a simulation platform capable of rendering high-quality synthetic sensor data and modeling real-world physics relevant to a variety of industries.
“There is a tendency to get caught up in how beautiful the simulation looks since we see these visuals, and it’s very pleasing. What really matters is how the AI algorithms perceive these pixels. But beyond the appearance, there are at least two other major aspects which are crucial to mimicking reality in a simulation.”
“We should think of simulation as an accelerator to what we do in the real world. It can save time and money and help us with a diversity of edge-case scenarios, but ultimately it is a tool to augment datasets collected from real-world data collection,” he said.