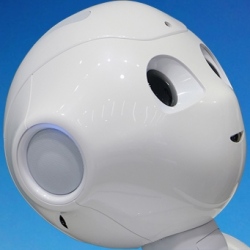
DARPA wants to have artificial intelligences with the capability of helping humans to trace the conclusions, decisions and reasoning of the AI. Success in machine learning has led to an explosion of new and cheap capabilities. Advances promise to produce yet more autonomous systems that perceive, learn, decide, and act on their own.
These systems offer tremendous benefits, but their effectiveness will be limited by the machine’s inability to explain its decisions and actions to human users. This issue is especially important for the Department of Defense (DoD), which is facing challenges that demand the development of more intelligent, autonomous, and symbiotic systems. Explainable AI will be essential if users are to understand, appropriately trust, and effectively manage this incoming generation of artificially intelligent partners.
The problem of explainability is, to some extent, the result of AI’s success.
In the early days of AI, the predominant reasoning methods were logical and symbolic. These early systems reasoned by performing some form of logical inference on (somewhat) human readable symbols. Early systems could generate a trace of their inference steps, which then became the basis for explanation. As a result, there was significant work on how to make these systems explainable.
DARPA is interested in creating technology to make this new generation of AI systems explainable. Because the most critical and most opaque components are based on machine learning, XAI (eXplainable Artificial Intelligence) is focusing on the development of explainable machine learning techniques. By creating new machine learning methods to produce more explainable models and combining them with explanation techniques, XAI aims to help users understand, appropriately trust, and effectively manage the emerging generation of AI systems.
The target of XAI is an end user who depends on decisions, recommendations, or actions produced by an AI system, and therefore needs to understand the rationale for the system’s decisions. For example, an intelligence analyst who receives recommendations from a big data analytics algorithm needs to understand why the algorithm has recommended certain activity for further investigation. Similarly, a test operator of a newly developed autonomous system will need to understand why the system makes its decisions so that he/she can decide how to use it in future missions.