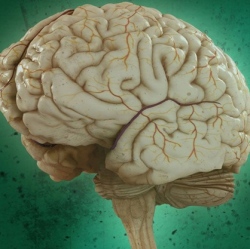
Machine learning is an extremely clever approach to computer programming. Instead of a having to carefully write out instructions for a particular task, you just feed millions of examples into a very powerful computer and, essentially, let it write the program itself.
A new $12 million dollar project at Carnegie Mellon University could make machine learning even more powerful by uncovering ways to teach computers more efficiently while using much less data.
The five-year effort will use a newish technique, called 2-photon calcium imaging microscopy, to study the way visual information is processed in the brain. The funding comes through President Obama’s BRAIN Initiative, and it is a good example of one of the near-term benefits that powerful new brain imaging techniques could have.
Many of the best machine-learning algorithms are, in fact, already loosely based on the functioning of the brain. But these are incredibly crude, and do not account for some simple features of biological networks.
“Powerful as they are, [these algorithms] aren’t nearly as efficient or powerful as those used by the human brain,” said Tai Sing Lee, a professor of computer science at CMU who is leading the effort. “For instance, to learn to recognize an object, a computer might need to be shown thousands of labeled examples and taught in a supervised manner, while a person would require only a handful and might not need supervision.”
It isn’t just neuroscience that could help us develop better machine-learning approaches. Some cognitive scientists are taking inspiration from psychology observations in order to build clever new learning systems.