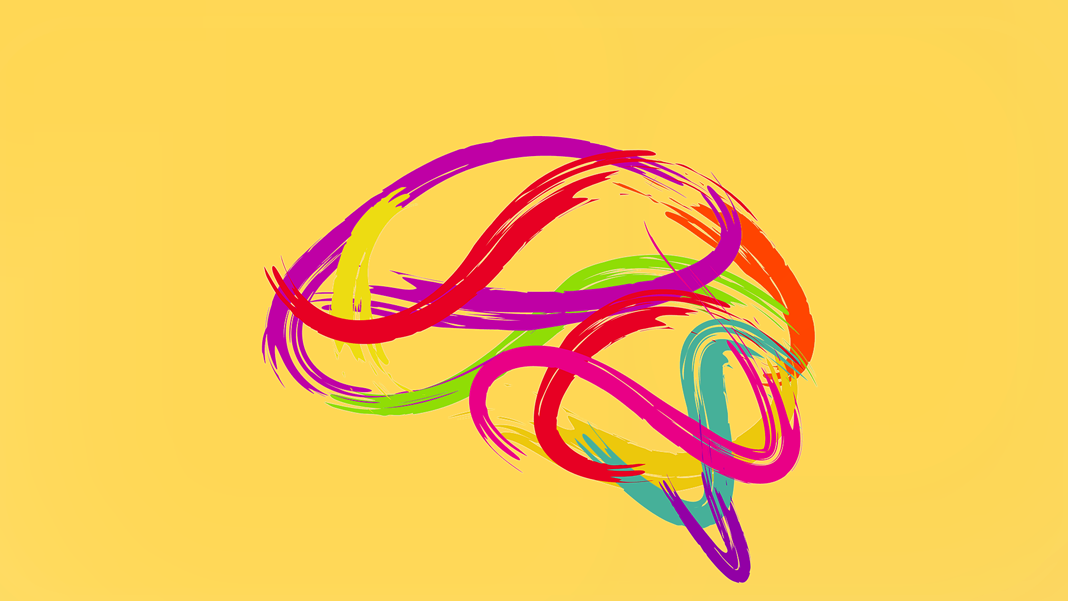
More speculatively, it also raises concerns around whether we would be able to detect dangerous behaviors, such as deception or power seeking, in more powerful future AI models.
A team from Anthropic has made a significant advance in our ability to parse what’s going on inside these models.
They’ve shown they can not only link particular patterns of activity in a large language model to both concrete and abstract concepts, but they can also control the behavior of the model by dialing this activity up or down.
The research builds on years of work on “Mechanistic interpretability,” where researchers reverse engineer neural networks to understand how the activity of different neurons in a model dictate its behavior.
That’s easier said than done because the latest generation of AI models encode information in patterns of activity, rather than particular neurons or groups of neurons.
The researchers had previously shown they could extract activity patterns, known as features, from a relatively small model and link them to human interpretable concepts.
The team decided to analyze Anthropic’s Claude 3 Sonnet large language model to show the approach could work on commercially useful AI systems.
The team says this suggests that the way ideas are encoded in these models corresponds to our own conceptions of similarity.
Massively amplifying the feature for the Golden Gate Bridge led the model to force it into every response no matter how irrelevant, even claiming that the model itself was the iconic landmark.
In one, they found that over-activating a feature related to spam emails could get the model to bypass restrictions and write one of its own.
The team say there’s little danger of attackers using the approach to get models to produce unwanted or dangerous output, mostly because there are already much simpler ways to achieve the same goals.
Turning the activity of different features up or down could also be a way to steer models towards desirable outputs and away from less positive ones.
The researchers were keen to point out that the features they’ve discovered make up just a small fraction of all of those contained within the model.
What’s more, extracting all features would take huge amounts of computing resources, even more than were used to train the model in the first place.