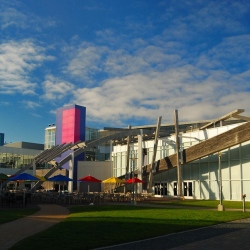
Making sense of the enormous amount of data that encodes human life remains a formidable challenge. But it is also precisely the sort of problem that machine learning excels at. Google released a tool called DeepVariant that uses the latest AI techniques to build a more accurate picture of a person’s genome from sequencing data.
DeepVariant helps turn high-throughput sequencing readouts into a picture of a full genome. It automatically identifies small insertion and deletion mutations and single-base-pair mutations in sequencing data.
High-throughput sequencing became widely available in the 2000s and has made genome sequencing more accessible. But the data produced using such systems has offered only a limited, error-prone snapshot of a full genome. It is typically challenging for scientists to distinguish small mutations from random errors generated during the sequencing process, especially in repetitive portions of a genome. These mutations may be directly relevant to diseases such as cancer.
A number of tools exist for interpreting these readouts, including GATK, VarDict, and FreeBayes. However, these software programs typically use simpler statistical and machine-learning approaches to identifying mutations by attempting to rule out read errors.
“One of the challenges is in difficult parts of the genome, where each of the [tools] has strengths and weaknesses,” says Brad Chapman, a research scientist at Harvard’s School of Public Health who tested an early version of DeepVariant. “These difficult regions are increasingly important for clinical sequencing, and it’s important to have multiple methods.”
DeepVariant was developed by researchers from the Google Brain team, a group that focuses on developing and applying AI techniques, and Verily, another Alphabet subsidiary that is focused on the life sciences.
The team collected millions of high-throughput reads and fully sequenced genomes from the Genome in a Bottle (GIAB) project, a public-private effort to promote genomic sequencing tools and techniques. They fed the data to a deep-learning system and painstakingly tweaked the parameters of the model until it learned to interpret sequenced data with a high level of accuracy.
Last year, DeepVariant won first place in the PrecisionFDA Truth Challenge, a contest run by the FDA to promote more accurate genetic sequencing.
“The success of DeepVariant is important because it demonstrates that in genomics, deep learning can be used to automatically train systems that perform better than complicated hand-engineered systems,” says Brendan Frey, CEO of Deep Genomics.
The release of DeepVariant is the latest sign that machine learning may be poised to boost progress in genomics.
Deep Genomics is one of several companies trying to use AI approaches such as deep learning to tease out genetic causes of diseases and to identify potential drug therapies (see “An AI-Driven Genomics Company Is Turning to Drugs”).
Frey says AI will eventually go well beyond helping to sequence genomic data. “The gap that is currently blocking medicine right now is in our inability to accurately map genetic variants to disease mechanisms and to use that knowledge to rapidly identify life-saving therapies,” he says.
Another prominent company in this area is Wuxi Nextcode, which has offices in Shanghai, Reykjavik, and Cambridge, Massachusetts. Wuxi Nextcode has amassed the world’s largest collection of fully sequenced human genomes, and the company is investing heavily in machine-learning methods.
DeepVariant will also be available on the Google Cloud Platform. Google and its competitors are furiously adding machine-learning features to their cloud platforms in an effort to lure anyone who might want to tap into the latest AI techniques (see “Ambient AI Is About to Devour the Software Industry”).
In general, AI figures to help many aspects of medicine take big leaps forward in the coming years. There are opportunities to mine many different kinds of medical data—from images or medical records, for example— to predict ailments that a human doctor might miss (see “The Machines Are Getting Ready to Play Doctor” and “A New Algorithm for Palliative Care”).
But genomic medicine represents an especially big opportunity, because the scale and complexity of the data is unprecedented. “For the first time in history, our ability to measure our biology, and even to act on it, has far surpassed our ability to understand it,” says Frey. “The only technology we have for interpreting and acting on these vast amounts of data is AI. That’s going to completely change the future of medicine.”