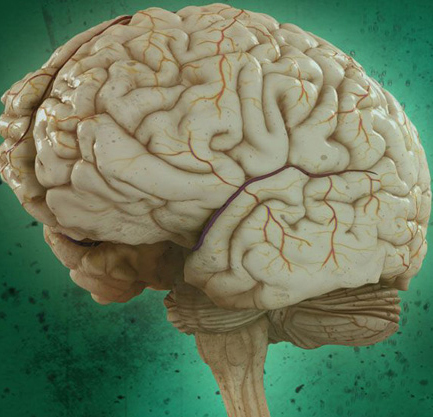
Scientists have long thought to decode this linguistic degeneration as an early indicator of Alzheimer’s. One idea is to use natural language software as a “Guide” of sorts that hunts down unusual use of language.
It seems obvious, but it’s a giant difficulty for AI. Our speech patterns, cadence, tone, and word choice are all colored with shades of personal history and nuances that the average language AI struggles to decipher. So why not tap into the most creative AI language tools today?
Using a publicly available dataset of speech transcripts from people with and without Alzheimer’s, the team retrained GPT-3 to pick out linguistic nuances that suggest dementia.
When fed with new data, the algorithm reliably detected Alzheimer’s patients from healthy ones and could predict the person’s cognitive testing score-all without any additional knowledge of the patients or their history.
“The use of speech as a biomarker provides quick, cheap, accurate, and non-invasive diagnosis of AD and clinical screening.”
Despite science’s best efforts, Alzheimer’s is incredibly hard to diagnose. The most insidious part of Alzheimer’s is that it’s hard to diagnose. Here’s the silver lining: even without a cure, diagnosing Alzheimer’s early can help patients and their loved ones make plans around support, mental health, and finding treatments to manage symptoms.
With the FDA’s recent approval of Leqembi, a drug that moderately helps protect cognitive decline in people with early-stage Alzheimer’s, the race to catch the disease early is heating up.
Rather than focusing on brain scans or blood biomarkers, the Drexel team turned to something remarkably effortless: speech.
“We know from ongoing research that the cognitive effects of Alzheimer’s disease can manifest themselves in language production,” said study author Dr. Hualou Liang.
“The most commonly used tests for early detection of Alzheimer’s look at acoustic features, such as pausing, articulation, and vocal quality, in addition to tests of cognition.”
Natural Language Processing has dominated the AI sphere in its ability to recognize everyday language.
By feeding it recordings of a patient’s voice or their writings, neuroscientists could highlight particular vocal “Tics” that a certain group of people may have-for example, those with Alzheimer’s.
In contrast, large language models, which underlie GPT-3, are far more flexible to provide a “Powerful and universal language understanding and generation,” the authors said.
“GPT-3’s systemic approach to language analysis and production makes it a promising candidate for identifying the subtle speech characteristics that may predict the onset of dementia,” said study author Felix Agbavor.
“Training GPT-3 with a massive dataset of interviews-some of which are with Alzheimer’s patients-would provide it with the information it needs to extract speech patterns that could then be applied to identify markers in future patients.”
The team readily used GPT-3 for two critical measures of Alzheimer’s: discerning an Alzheimer’s patient from a healthy one and predicting a patient’s severity of dementia based on a benchmark for cognition dubbed the Mini-Mental State Exam.
Here, the team fed it the ADReSSo Challenge, which contains everyday speech from people with and without Alzheimer’s.
For the first challenge, the team pitted their GPT-3 programs against two that hunt down specific “Tics” in language. The algorithms fared even better when predicting the accuracy of the dementia MMSE by speech features alone. When pitted against other state-of-the-art Alzheimer’s detection models, the Babbage edition crushed the opponents for accuracy and level of recall.
As this technology develops, it might become a crucial tool to help doctors make better diagnoses.