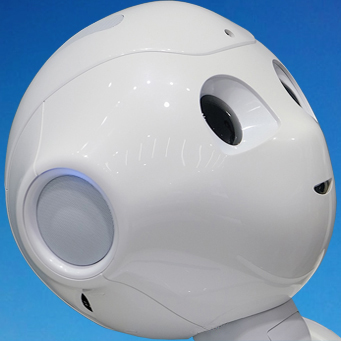
The dream of a robot butler seems tantalizingly close, but first we need to teach them how to navigate our cluttered human environments. That’s why Facebook has created an ultra-realistic simulator to train AI to carry out our bidding in a variety of indoor settings.
Virtual environments from Atari videogames to self-driving car simulators have emerged as a leading tool for teaching machines how to explore and interact with their environments. One of the main reasons is that they give us complete control over the agent’s environment. The real world is a messy place with too many variables to account for, but in a simulation not only do we know all the variables, we can tweak them to suit our experiment.
But that comes at a cost. The tightly-controlled world of a simulation is very different from the environment a physical robot will find itself in, with all kinds of challenges from lighting to weather to unpredictable humans. AI that performs flawlessly in virtual environments often flops in the real world.
Training AI in the real world is impractical, though, because our most advanced machine learning approaches are data-hungry, so agents have to practice thousands or millions of times to get it right. Virtual environments can be sped up hundreds of times faster than real time, and running hundreds of experiments in parallel just requires a few more computer chips rather than hundreds of new robots and test environments.
An emerging solution to this problem is to create ever more realistic digital environments. The idea is that the closer we get to the real world in simulation, the easier it will be for AI to transfer what it learns to the real world. This is the logic behind Facebook’s new Replica dataset, which provides a photorealistic 3D mirror of various indoor environments, including an apartment and a retail store.
The data was captured directly from the real world using a specially-designed camera rig that combines HD video, high-precision depth data, and a simultaneous localization and mapping (SLAM) system that creates a record of where every measurement was taken from.
The approach has managed to capture things like reflective surfaces and complex textures that are often difficult to reproduce. On top of this, Facebook engineers have meticulously labeled everything in the dataset, including color coding different categories of objects.
All this data can then be loaded into Facebook’s Habitat simulator platform—which also runs other 3D simulations like Gibson and Matterport3D—to create a 3D environment for AI to explore.
As well as helping to train AI to navigate, the company also hopes the dataset can improve augmented reality (AR) technology. The idea is that giving AI a better understanding of the physical spaces humans occupy could help it better mesh the digital projections of AR with the real world. For instance, ensuring that while you’re chatting with a 3D virtual avatar of your grandmother, she doesn’t keep walking through the back of the couch.
The company has open-sourced both Habitat and the Replica dataset so anyone can use the powerful simulations to train their AI. Nonetheless, Replica does still have some gaps that will limit the ability to transfer lessons learned straight into the real world.