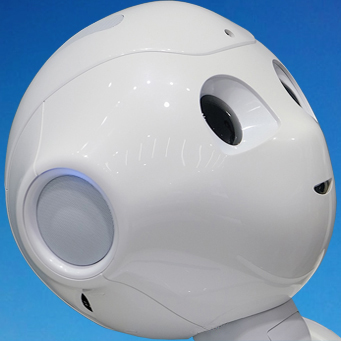
Human success is rooted in teamwork, and recent advancements suggest that AI could benefit similarly from collaboration. Despite the impressive capabilities of large language models (LLMs), companies have yet to find a transformative application for widespread AI adoption.
One emerging use case is autonomous AI agents, though their error-prone nature limits their reliability for complex tasks.
Research into “multi-agent systems” demonstrates that collaborating chatbots can overcome individual limitations and tackle tasks beyond the reach of single AIs.
This field gained momentum when Microsoft released AutoGen, a software library facilitating the creation of LLM teams. AutoGen allows multiple LLM-powered agents to communicate using natural language, simplifying the development of AI teams.
Several promising studies presented at the International Conference on Learning Representations (ICLR) highlight the potential of multi-agent systems. For example, AI collaboration improved performance on math tasks and enhanced reasoning and factual accuracy. In another scenario, three LLM-powered agents defused virtual bombs more efficiently than solo agents, with one agent even taking on a leadership role.
Chi Wang, leading the AutoGen project, notes that this approach leverages the ability to break tasks into smaller components, enabling parallel processing by AI teams. However, setting up these teams has traditionally been complex and accessible only to AI researchers. To address this, Microsoft launched AutoGen Studio, a “low-code” interface for building AI teams, making it accessible to non-experts. Users can select preset AI agents or customize their own by choosing LLMs, assigning skills, and writing prompts.
AutoGen Studio users have employed AI teams for various tasks, including travel planning, market research, data extraction, and video generation. However, challenges remain. Running multiple LLMs is costly, and it is uncertain if AI teams are more robust against mistakes or prone to cascading errors. Additionally, practical issues like structuring AI teams, distributing responsibilities, and integrating AI with human teams need further exploration. Despite these challenges, pooling AI resources is a promising and rapidly evolving concept.