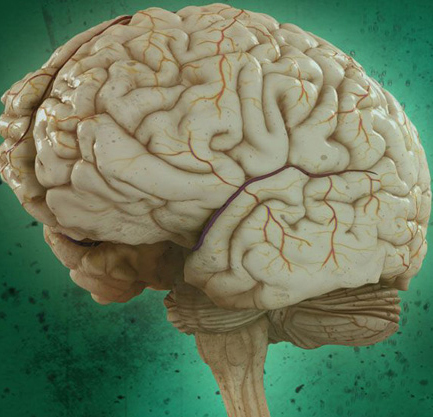
There’s no doubt that deep learning-a type of machine learning loosely based on the brain-is dramatically changing technology.
From predicting extreme weather patterns to designing new medications or diagnosing deadly cancers, AI is increasingly being integrated at the frontiers of science.
Deep learning has a massive drawback: The algorithms can’t justify their answers.
For now, deep learning-based algorithms-even if they have high diagnostic accuracy-can’t provide that information.
In a study in Nature Computational Science, they combined principles from the study of brain networks with a more traditional AI approach that relies on explainable building blocks.
Dubbed “Deep distilling,” the AI works like a scientist when challenged with a variety of tasks, such as difficult math problems and image recognition.
“Deep distilling is able to discover generalizable principles complementary to human expertise,” wrote the team in their paper.
The barrier for most deep learning algorithms is their inexplicability.
Compared to deep learning, symbolic models are easier for people to interpret.
The team was inspired by connectomes, which are models of how different brain regions work together.
In several tests, the “Neurocognitive” model beat other deep neural networks on tasks that required reasoning.
This process is what leads to new materials and medications, deeper understanding of biology, and insights about our physical world.
Deep learning has been especially useful in the prediction of protein structures, but its reasoning for predicting those structures is tricky to understand.
Called deep distilling, the AI groups similar concepts together, with each artificial neuron encoding a specific concept and its connection to others.
Trained on simulated game-play data, the AI was able to predict potential outcomes and transform its reasoning into human-readable guidelines or computer programming code.
Deep distilling could be a boost for physical and biological sciences, where simple parts give rise to extremely complex systems.
The new study goes “Beyond technical advancements, touching on ethical and societal challenges we are facing today.” Explainability could work as a guardrail, helping AI systems sync with human values as they’re trained.
That’s the next step in deep distilling, wrote Bakarji.