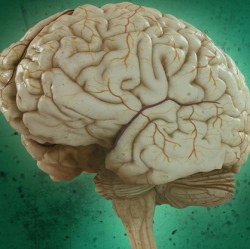
Noninvasive brain–computer interface (BCI) systems can restore functions lost to disability — allowing for spontaneous, direct brain control of external devices without the risks associated with surgical implantation of neural interfaces.
But as machine-learning algorithms have become faster and more powerful, researchers have mostly focused on increasing performance by optimizing pattern-recognition algorithms.
But what about letting patients actively participate with AI in improving performance?
To test that idea, researchers at the École Polytechnique Fédérale de Lausanne (EPFL), based in Geneva, Switzerland, conducted research using “mutual learning” between computer and humans — two severely impaired (tetraplegic) participants with chronic spinal cord injury. The goal: win a live virtual racing game at an international event.
The participants were trained to improve control of an avatar (a person-substitute shown on a computer screen) in a virtual racing game. The experiment used a brain-computer interface (BCI), which uses electrodes on the head to pick up control signals from a person’s brain.
Each participant (called a “pilot”) controlled an on-screen avatar in a three-part race. This required mastery of separate commands for spinning, jumping, sliding, and walking without stumbling.
After training for several months, in Oct. 8, 2016, the two pilots participated (on the “Brain Tweakers” team) in Cybathlon in Zurich, Switzerland, the first international para-Olympics for disabled individuals in control of bionic assistive technology.*
The BCI-based race consisted of four brain-controlled avatars competing in a virtual racing game called “Brain Runners.” To accelerate each pilot’s avatar, they had to issue up to three mental commands (or intentional idling) on corresponding color-coded track segments.
The two participants in the EPFL research had the best three times overall in the competition. One of those pilots won the gold medal and the other held the tournament record.
The researchers believe that with the mutual-learning approach, they have “maximized the chances for human learning by infrequent recalibration of the computer, leaving time for the human to better learn how to control the sensorimotor rhythms that would most efficiently evoke the desired avatar movement.
Our results showcase strong and continuous learning effects at all targeted levels, machine, subject, and application, with both [participants] over a longitudinal study lasting several months,” the researchers conclude.