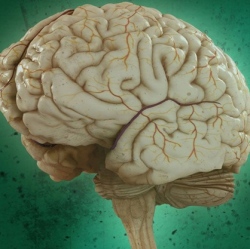
Numenta have published a new theory that represents a breakthrough in understanding how networks of neurons in the neocortex learn sequences. A paper, ‘Why Neurons Have Thousands of Synapses, A Theory of Sequence Memory in Neocortex,” has been published in the Frontiers in Neural Circuits Journal.
“This study is a key milestone on the path to achieving that long-sought goal of creating truly intelligent machines that simulate human cerebral cortex and forebrain system operations,” commented Michael Merzenich, PhD, Professor Emeritus UCSF, Chief Scientific Officer for Posit Science.
The Numenta paper introduces two advances: First, it provides an explanation of why neurons in the neocortex have thousands of synapses, and why the synapses are segregated onto different parts of the cell, called dendrites. The authors propose that the majority of these synapses are used to learn transitions of patterns, a feature missing from most artificial neural networks.
Second, the authors show that neurons with these properties, arranged in layers and columns, a structure observed throughout the neocortex – form a powerful sequence memory. This suggests the new sequence memory algorithm could be a unifying principle for understanding how the neocortex works.
Through simulations, the authors show the new sequence memory exhibits a number of important properties such as the ability to learn complex sequences, continuous unsupervised learning, and extremely high fault tolerance.
“Our paper makes contributions in both neuroscience and machine learning,” Hawkins noted. “From a neuroscience perspective, it offers a computational model of pyramidal neurons, explaining how a neuron can effectively use thousands of synapses and computationally active dendrites to learn sequences. From a machine learning and computer science perspective, it introduces a new sequence memory algorithm that we believe will be important in building intelligent machines.”
“This research extends the work Jeff first outlined in his 2004 book On Intelligence and encompasses many years of research we have undertaken here at Numenta,” said Ahmad, “It explains the neuroscience behind our HTM (Hierarchical Temporal Memory) technology and makes several detailed predictions that can be experimentally verified. The software we have created proves that the theory actually works in real world applications.”
Numenta’s primary goal is to reverse engineer the neocortex, to understand the detailed biology underlying intelligence. The Numenta team also believes this is the quickest route to creating machine intelligence. As a result of this approach, the neuron and network models described in the new paper are strikingly different than the neuron and network models being used in today’s deep learning and other artificial neural networks.
Functionally, the new theory addresses several of the biggest challenges confronting deep learning today, such as the lack of continuous and unsupervised learning.