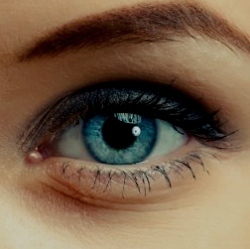
As the cost of sequencing DNA goes down more and more, people’s genome data is being collected for research and personalized medicine. This data is often anonymized by decoupling it from other identifying information, but a biotech company says it can use your genome to build a computer-generated image of your face.
The claims have been hotly contested, but the authors of the paper say the results could have both potential law enforcement applications and significant privacy implications.
Being able to generate mugshots of suspects from DNA evidence found at the scene of a crime could be incredibly useful for police, but it’s also conceivable the same approach could be used to identify people who have anonymously donated their DNA to research databases.
This could be used for anything from identity theft to medical insurers trying to tailor individual premiums based on people’s genetic propensity for certain diseases.
The research was conducted by Human Longevity Inc (HLI), which is led by scientist and entrepreneur J. Craig Venter. He rose to fame at the turn of the century as the head of Celera Genomics, the private company that raced the publicly-funded Human Genome Project to sequence the human genome.
Since then he’s become a poster boy for synthetic biology, creating what he and colleagues at his J. Craig Venter Institute claimed was the first synthetic life in 2010. He co-founded HLI in 2013 to create the world’s largest database of human genotypes and phenotypes, use machine learning to uncover insights into the genetic basis of disease, and offer personalized medicine services.
In a paper in the Proceedings of the National Academy of Sciences published last week, Venter and colleagues have claimed that their algorithms are capable of using people’s genome data to identify them. The system also outputs computer-generated images supposed to represent the subject’s face.
The group sequenced the genomes of 1,061 volunteers from diverse ethnic backgrounds. They also collected biometric data such as their height, weight, eye color, 3D scans of their faces, and recordings of their voices, as well as demographic data like age, self-reported gender, and ethnicity.
They then trained a series of predictive models on this data and the genomic data. The models for traits with simple and obvious genetic markers like eye color, skin color, and sex were relatively accurate, but traits with more complicated genetic underpinnings proved more difficult.
However, they then combined all of these predictions into a single machine learning algorithm. Using this combined prediction, in an ethnically mixed group the researchers claimed to be able to re-identify an average of eight out of ten people. However, this fell to just five out of ten when the group was restricted to a single ethnicity.
That last point is one of the sources of criticism of the paper. Former employee Jason Piper, who left the company 12 months ago but is registered as an author on the paper, took to Twitter soon after publication to say that most of the predicted faces look very similar and are effectively just average faces for a particular race. “Because everyone looks close to the average of their race, everyone looks like their prediction!” he tweeted.
Elsewhere, in a rebuttal uploaded to the pre-print server bioArxiv, Columbia University computer scientist and chief scientific officer of genealogy website MyHeritage.com Yaniv Erlich claimed he achieved similar results on the same dataset by simply comparing genome data against the demographic measures of age, sex, and self-reported ethnicity.
“The take-home message should be that identifying someone in a group of ten people requires very little effort,” he wrote. “Anyone with access to even low dimensional data, such as basic demographic, can do that.”
Regardless of the merits of this paper, though, as the authors point out, the predictive performance of this kind of approach is only likely to improve with larger sample sizes and as large genome sequencing efforts teach us more about the links between genotype and phenotype.
“We believe that as we increase the numbers of people in this study and in the HLI database to hundreds of thousands we will be able to accurately predict all that can be predicted from individuals’ genomes,” Venter said in a press release.
“We are also concerned that the public and the research community at large are not adequately focused on the need for better safeguards and policies for individual privacy in the genomics era and are urging more analysis, better technical solutions, and continued discussion.”
A report in MIT Tech Review notes that this concern about privacy, particularly when it comes to the research community, which tends to share data, could be seen as self-serving considering HLI is amassing a private and therefore possibly more secure database of its own.
But it’s hard to argue with the logic. Our DNA, to a large extent, determines who we are and what we look like, so whether or not this particular approach is capable of identifying us, it’s almost certain that advances in both genetics and data analytics will make it possible in the not-so-distant future.
The argument, then, is essentially about who you think you can trust with that data—governments or companies. The answer for most people will likely depend on which end of the political spectrum they fall.