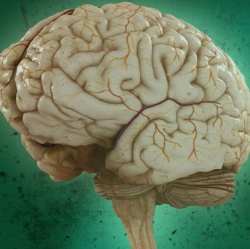
Northwestern Engineering have developed a model that could give computers the ability to reason more like humans. With a structure mapping engine (SME), the new model is capable of analogical problem solving, including capturing the way humans use analogies between situations to solve moral dilemmas.
“In terms of thinking like humans, analogies are where it’s at,” said Forbus, Walter P. Murphy Professor of Electrical Engineering and Computer Science in Northwestern’s McCormick School of Engineering. “Humans use relational statements fluidly to describe things, solve problems, indicate causality, and weigh moral dilemmas.”
The theory underlying the model is psychologist Dedre Gentner’s structure-mapping theory of analogy and similarity, which has been used to explain and predict many psychology phenomena. Structure-mapping argues that analogy and similarity involve comparisons between relational representations, which connect entities and ideas, for example, that a clock is above a door or that pressure differences cause water to flow.
Previous models of analogy, including prior versions of SME, have not been able to scale to the size of representations that people tend to use. The inherent complexity of the arguments have not been able to be realized in artificial intelligence algorithms. Now, Forbus’s new version of SME can handle the size and complexity of relational representations that are needed for visual reasoning, cracking textbook problems, and solving moral dilemmas.
“Relational ability is the key to higher-order cognition,” said Gentner, Alice Gabrielle Twight Professor in Northwestern’s Weinberg College of Arts and Sciences. “Although we share this ability with a few other species, humans greatly exceed other species in ability to represent and reason with relations.”
Artificial intelligence systems, like Google’s AlphaGo, rely on deep learning, a process in which a computer learns examining massive amounts of data. By contrast, people, and SME-based systems, often learn successfully from far fewer examples. In moral decision-making, for example, a handful of stories suffices to enable an SME-based system to learn to make decisions as people do in psychological experiments.
“Given a new situation, the machine will try to retrieve one of its prior stories, looking for analogous sacred values, and decide accordingly,” Forbus said.
SME has also been used to learn to solve physics problems from the Advanced Placement test, with a program being trained and tested by the Educational Testing Service. As further demonstration of the flexibility of SME, it also has been used to model multiple visual problem-solving tasks.
Forbus’s team is releasing the SME source code and a 5,000-example corpus, which includes comparisons drawn from visual problem solving, textbook problem solving, and moral decision making. The goal of this is to spur on further research in the area.
So far, the range of tasks successfully tackled by SME-based systems suggests that analogy might lead to a new technology for artificial intelligence systems as well as a deeper understanding of human cognition.
In one example, analogy-based artificial intelligence techniques could be valuable across a range of applications, including security, health care, and education.
“SME is already being used in educational software, providing feedback to students by comparing their work with a teacher’s solution,” Forbus said. "But there is a vast untapped potential for building software tutors that use analogy to help students learn.”